To tackle several cybersecurity challenges, companies are now using AI and data analytics to gain an advantage
Cyberattacks are a growing hazard in today’s data-centric environments, targeting multiple threat surfaces parallelly using a broad range of techniques to evade detection and access valuable data. The most-used attack strategies are social engineering, phishing, ransomware, and malware to gain privileged access credentials to bypass Identity Access Management (IAM) and Privileged Access Management (PAM) systems.
Once they enter a network, bad actors can move laterally across an organisation, searching for the most valuable data available to exfiltrate, sell, or impersonate senior executives. A 2021 research by IBM found that it takes an average of 287 days to identify and contain a data breach, with an average cost of $3.61 million in a hybrid cloud environment. When ransomware is used as the attack strategy, the average cost of a data breach skyrockets to $4.62 million.
Companies are now using AI and data analytics to gain an advantage, alerting beforehand to tackle such challenges.
Using AI As A Prevention Method
Cyberattack prevention is a perfect use case for AI, and machine learning (ML), aiding to decipher millions of concurrent data connections typically present in an enterprise with the outside world. Training supervised ML algorithms with active data streams help them identify potential anomalies, even before the algorithm understands the definition of an anomaly.
Transaction fraud detection is one top priority amongst the five core areas where AI and ML can help cybersecurity improve this year. Additionally, malware detection and machine behavioural analysis are also in the top five use cases with the most value based on their exponential use of AI and ML in 2022.
Boston Consulting Group and Capgemini Research Institute report compares AI use cases in cybersecurity, its complexity and its benefits. Cybersecurity vendors whose platforms lie in the “high benefits, high complexity” quadrant are considered the best equipped, using AI and ML to lure attackers into simulated honeytraps, aiding to reverse engineer payloads, often down the executable file level.
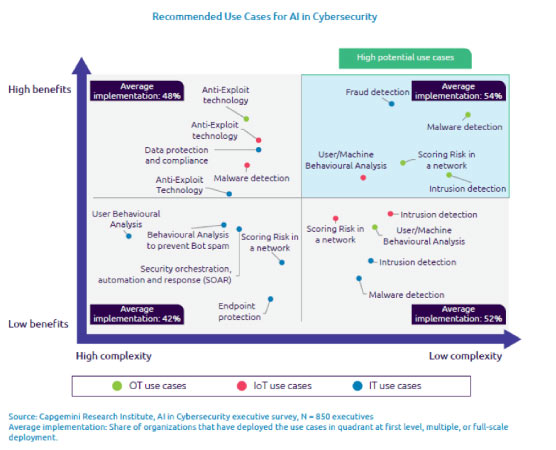
How AI Can Improve Current Systems
While the apps and platforms for cyberattack prevention already include advanced analytics and detailed modelling, the complete feature set rarely gets used. Enterprises now see AI and ML cyber security-based systems as relief for their overwhelmed staff. According to BCG, 56 per cent of executives say their cybersecurity analysts are currently overwhelmed. CISOs are now taking a more pragmatic view of AI and ML’s potential contributions to their operations, as they focus more on better protecting machine-based transactions.
Machine-based transaction attacks concern CISOs and their teams most because of their rapid nature and difficulty in identifying, predicting, and stopping. BCG found that 43% of executives saw an increase in machine-speed attacks. With seven out of every ten executives believing they cannot respond or prevent advanced cyberattacks without using AI as a defence, the demand for AL and ML-based cybersecurity systems in the following five core areas continues to grow.
Pandemic’s effects on eCommerce sales is one of the driving forces for investing in AI and ML-based transaction fraud detection. Transaction fraud detection is specially designed to provide real-time monitoring of payment transactions and uses ML techniques to identify anomalies and potentially evolving fraud attempts. In addition, ML algorithms are being trained more to identify different login processes and prevent account takeovers (ATOs), which is one of the fastest-growing online retail frauds today.
Organisations fell victim to a ransomware attack every 11 seconds in 2021, up from 40 seconds. AI and ML provide an edge against ransomware by automating patch management with AI bots, instead of relying on traditional brute-force inventory methods for improving the endpoints.
AI-powered bots use constraint-based algorithms to analyse and pinpoint which endpoints need updates and probable risk levels. Such algorithms use current and historical data to identify the specific patch updates and provide the build with any given endpoint device needs.
Another advantage of taking a bot-based approach to patch management is how it can autonomously scale across all endpoints and networks of an organisation. Automated patch management systems require more historical ransomware data to train AI and ML-based models better and fine-tune their predictive accuracy further.
AL and ML are also paying off in process behaviour analysis due to their combined strengths for quickly identifying potential breach attempts and acting rapidly on them. Process behaviour analysis concentrates on identifying anomalous, potentially malicious behaviour earlier based on patterns in behaviour.
As a result, it has proven particularly effective in several attacks that do not necessarily carry payloads. An excellent example of process behaviour analysis is how the Microsoft Defender 365 relies on behaviour-based detections, inculcating ML to identify when endpoints need to be healed and carry out the necessary steps autonomously with no human interaction. A virtual analyst is also made present as a part of the Microsoft 365 Defender suite that provides independent investigation and response.
Improving cybersecurity from endpoint to the core of IT infrastructures needs to be every enterprise’s goal. AI and ML have shown their proven potential in five core areas to improve cybersecurity, ransomware attempts, and learn from multiple data patterns to predict potential attack scenarios and vectors.
Attacks are more orchestrated today than ever before, often relying on machine-to-machine communication. Analysis through AI and ML stand the best chance of keeping up with cyberattack attempts while also increasing the innovation towards outsmarting attackers who are constantly evolving and stepping up their efforts.
If you liked reading this, you might like our other stories
Top 10 Endpoint Security Providers
How AI Is Making Banks Cost-Effective and Efficient