AI algorithms can potentially be misused for malicious purposes, a massive hindrance to AI/ML growth
Artificial Intelligence (AI) is a rapidly growing technology that has served many benefits to society. But since its advent, AI has been met with several ethical criticisms, which sparked a common hesitation among industries for fully adopting it.
There is a growing concern that the vulnerabilities in machine learning models and AI algorithms can become a part of malicious purposes and potentially be misused, hindering AI/ML growth. One of the most troubling misuses of AI in recent times is adversarial AI attacks. AI researchers are now focusing on this crucial concern of machine learning, a data manipulation technique that causes trained models to behave in undesired ways.
How it occurs
During an adversarial AI attack, AI is used to manipulate or deceive another AI system maliciously. Most AI programs can either learn, adapt or evolve through behavioural learning. This sometimes leaves them vulnerable to exploitation because it creates space for anyone to imbed the AI algorithm with malicious actions, further leading to adversarial results. Cybercriminals and threat actors can easily exploit this vulnerability for malicious purposes and intent.
The basics of an adversarial attack are fundamentally similar to a cyber attack. However, an adversarial attack typically entails two main types – poisoning and contamination. In the process of poisoning, the ML model is fed with inaccurate or misinterpreted data to dupe it into making an erroneous prediction. For contamination, the ML model is fed with multiple data for deceiving a trained model to conduct wrong actions or predictions.
In between both methods, contamination can become a widespread threat, since the technique involves injecting or feeding negative information. In contrast, it is fairly easy to control and prevent poisoning, as providing a training dataset would require an insider job, and it is possible to prevent such insider threats using zero-trust security models and other network security protocols.
The magnitude of the threat
AI is a vital part of critical fields such as finance, healthcare and transportation and security issues in these vulnerable fields can be hazardous, wreaking massive havoc. An Office of the Director of National Security report highlights numerous adversarial machine learning threats. Amidst these threats listed in the report, one of the most pressing concerns was these attacks’ potential to compromise computer vision algorithms. Research has come across several examples of AI positioning. One study involved researchers adding small changes to an image of a panda that is almost invisible to the naked eye. These changes caused the ML algorithm to identify the image of the panda as that of a gibbon.
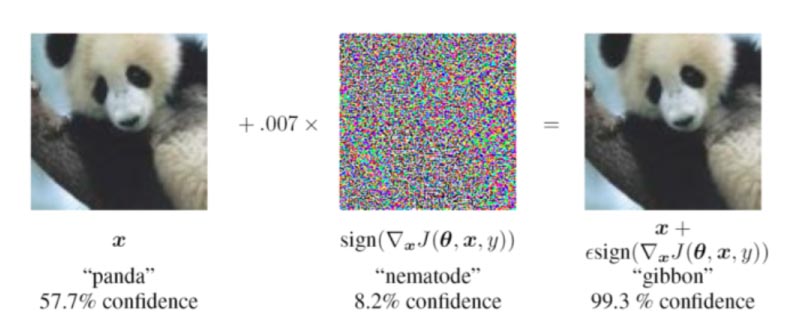
Similarly, another study highlighted the possibility of AI contamination which involved attackers duping the facial recognition cameras with infrared light. This allowed these attackers to mitigate accurate recognition and enable them to impersonate other people.
Prevention is better than cure
Researchers have been now devising multiple ways for protecting systems against adversarial AI. One such method is adversarial training, which involves pre-training a machine learning algorithm against positioning and contamination attempts, by feeding it with perturbations or small changes. The algorithm employs several non-intrusive image quality features to distinguish between legitimate and adversarial inputs. The technique can ensure that adversarial machine learning importer and alternation are neutralised before reaching the classification information. Another method includes pre-processing and denoising, which automatically removes possible adversarial noise from the input.
Although most adversarial attacks have been performed by researchers and within labs, they are a growing concern. The occurrence of adversarial attacks on AI or a machine learning algorithm now highlights a deep crack in the AI mechanism. Such vulnerabilities within the AI systems can stunt AI growth and development and become a significant security risk for people using AI-integrated systems. Therefore, to fully utilise the potential of AI systems and algorithms, it is crucial to understand and mitigate adversarial AI attacks.
If you liked reading this, you might like our other stories
Is AI Changing Content Marketing?
Is Ethical Hacking Our Last Defence?