Vision foundation models can work on multiple vision-related tasks simultaneously, including object recognition, picture categorisation, and visual-language understanding
Modern research is aiding the creation of AI systems that can learn from millions of examples to help us better understand our world and find practical solutions to complex problems. These large-scale models have led to systems that can understand when we talk or write, such as natural language processing or understanding behavioural requirements.
With growing developments in computer vision technology, object identification, recognition, and understanding of the context of items in a picture have become increasingly crucial for developing next-gen AI architectures. Recognition and analysis of complex images can sometimes lead to indecisive results, and finding solutions to these problems heightened interest in developing general-purpose vision systems.
General-purpose vision systems, often referred to as vision foundation models, work on multiple vision-related tasks simultaneously, including object recognition, picture categorisation, and visual-language (VL) understanding.
Understanding foundation models
The AI landscape is dominated by task-specific models. But intelligent AI systems with contextual understanding can provide solutions to various real-world issues with no human intervention by learning joint, fundamental representations that support a wide range of tasks. Foundation models can be used to train big data and create modifications to new bottlenecks. These models result from several deep learning and transfer learning constructions. To promote adaptability and provide a solid platform for future applications, a foundation model can act as a common ground for multiple task-specific models.
Due to their outstanding performance and generalisation abilities, foundation models have become increasingly popular. Researchers and developers worldwide use developed models widely in AI pipelines for NLP and computer vision systems since they can be swiftly incorporated and deployed into any real-world AI system.
Advancing vision through the foundation
The integration of localisation and understanding together in design has long been a challenge for systems. By doing this, pre-training procedures can be shortened, and expenses reduced. As opposed to VL understanding tasks, which emphasise the fusion of two modalities and call for a variety of high-level semantic outputs, localisation tasks emphasise the use of vision alone and need fine-grained output. Over the years, academics have tried to combine these tasks into a fundamental multi-task method. Two distinct high-level branches are made for localisation and VL comprehension, respectively, while a low-level visual encoder can be shared between tasks.
General-purpose vision systems or vision foundation models can simplify processing multiple vision tasks at once. Integrating localisation tasks like object recognition, segmentation, and VL understanding is particularly relevant for VQA and picture captions.
VL grounding, a technique that can improve both localisation and comprehension needs, is a crucial procedure in such structures. Two components of VL grounding are understanding input and identifying the objects mentioned in the image.
As a unified model for localisation and VL understanding tasks, a grounded VL understanding model helps in semantic classification processes, where classification can be framed as a VL understanding task using the classification-to-matching method. Localisation data is converted into VL grounding data as needed; hence, understanding data such as image-text pairings can be simply self-trained into VL grounding data. As a result, the AI achieves a unified pre-training procedure in which all task data are converted to grounding data, and contextual comprehension is received as output.
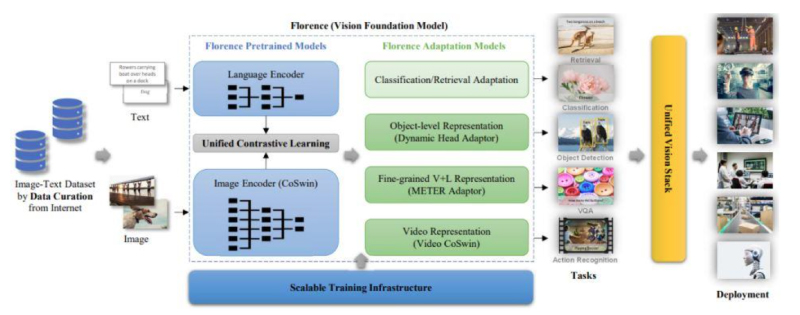
Modern developments
The Microsoft research team developed Florence, a novel foundation model for computer vision that has significantly outperformed previous large-scale pre-training approaches by achieving astonishing SOTA results across a variety of visual and visual-linguistic benchmarks. Florence possessed excellent generalisation skills and was trained on noisy web-scale data from beginning to end. The Florence ecosystem uses vast online visual data for data curation, model pre-training, task adaptations, and training infrastructure.
The researchers collected 900 million image-text pairs in a brand-new noisy web-crawled dataset. Florence’s effective real-world adaptation possible by few-shot and zero-shot transfer learning. For model pre-training, it employs a two-tower architecture made up of an image encoder and a language encoder that helps address the crucial challenge of task adaptation and learns feature representations along with space, time, and modality. The training infrastructure uses methods like ZeRO, activation checkpointing, mixed-precision training, and gradient cache to minimise memory processing dramatically.
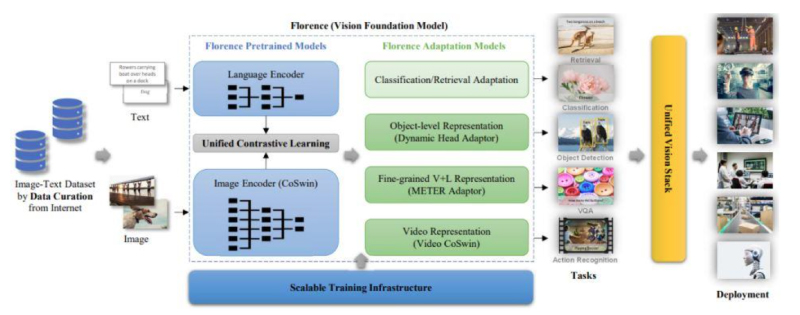
Recently, Microsoft unveiled the GLIPv2, which supports localisation and VL comprehension tasks, as another unified framework for vision-language representation learning. GLIPv2 exhibits enhanced zero-shot and few-shot transfer learning capabilities to open-world object recognition and instance segmentation tasks on the LVIS dataset and the “Object Detection in the Wild (ODinW)” benchmark, owing to its semantically rich annotations from image-text data. As a grounding model, it generates VL knowledge with great grounding capabilities that are self-explanatory and simple to debug.
The development of AI and computer science through foundation model applications is now in its early stages. The current uses for such models have the potential to change businesses and raise processing standards within industries. Foundation models can aid in developing a robust framework capable of scaling and adapting to a wide range of tasks and applications. With innovations in the domain of vision foundation, the future of computer vision seems exciting, and it will be interesting to watch tech behemoths compete in this new market.
If you liked reading this, you might like our other stories
The AI and Data-Driven BFSI Future
Harnessing The Power Of AI-driven XDR