The arrival of transformers suggested the possibility of convergence among contrasting subfields of AI, something that seemed nearly impossible a few years back
Before transformers became a highly used element of data science, the progress on AI-based language tasks developments in other areas lagged. Transformers quickly became the front-runner for several applications like word recognition, which focuses on analysing and predicting text. It also led to a wave of tools, like OpenAI’s Generative Pre-trained Transformer 3 (GPT-3). But what else could they do? The answer seems to be unfolding now, as researchers report that transformers are surprisingly versatile in several areas.
Transformers leading the charge
In vision-related tasks, such as image classification, neural nets that use transformers have become faster and more accurate. Emerging work in several other areas of AI, like processing a variety of multiple inputs at once or planning tasks, has shown that transformers can handle more load. The arrival of transformers also suggested the possibility of convergence among contrasting subfields of AI, which seemed nearly impossible a few years back.
One of the most promising steps toward discovering and expanding the range of transformers began months after the release of a research paper titled Attention Is All You Need. Alexey Dosovitskiy, a former computer scientist at Google Brain Berlin, worked on computer vision. Dosovitskiy was trying to solve one of the biggest challenges in the field, which was to scale up CNNs and train them on larger data sets that represented images in ever-higher resolution without piling on the processing time. The eventual result was a network dubbed the Vision Transformer, or ViT, which his research group proposed at a conference in May 2021.
The model’s architecture was nearly identical to that of the first transformer proposed in 2017, with only minor changes allowing it to analyse images instead of words. By processing pixels divided into groups and applying self-attention to each, the ViT model could process enormous training data sets, resulting in amazingly accurate classifications. The created transformer classified images with over 90 per cent accuracy, a far better result than Dosovitskiy expected, later propelling it to a top rank at the ImageNet classification challenge, a seminal image recognition contest.
ViT’s success showed that maybe convolutions are not as fundamental to computer vision as researchers believed. Additional results bolstered these predictions. Other researchers routinely tested their models for image classification on the ImageNet database. At the beginning of 2022, an updated version of ViT was put forward, an approach that combined CNNs with transformers.
Researchers are now applying transformers to an even more challenging task: inventing new images. In a paper presented last year, researchers combined two transformer models to produce unique images, a much more complicated problem. When trained on the faces of more than 200,000 celebrities, a double transformer network showed the ability to synthesise new facial images at moderate resolution. The invented celebrities are impressively realistic and highly convincing, created by CNNs.
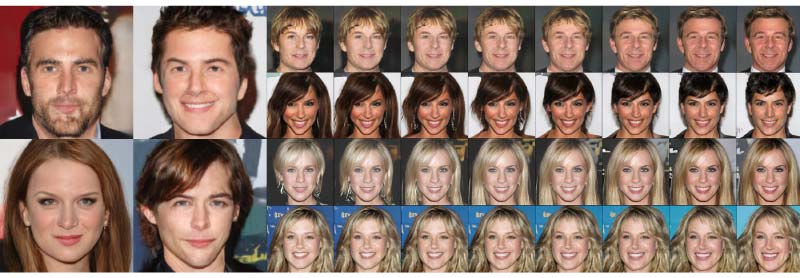
Cost of improvement?
Even if transformers unite and improve the tools of AI, emerging technologies come at a steep cost, and transformers seem to be no different. A transformer requires a higher outlay of computational power, in the pre-training phase itself, before beating the accuracy of its conventional competitors. This training expense could still be a drawback to the widespread implementation of transformers. Even though visual transformers have ignited newer efforts to push AI forward, many modern models still incorporate the best parts of convolutions. This means that the future models are more likely to use both than completely abandoning CNNs, and the prospect of hybrid AI architectures will depend on the strengths of transformers.
Emerging work suggests a spectrum of new uses for transformers in other AI domains, including teaching robots to recognise human body movements, training machines to discern emotions in speech, and detecting stress levels in electrocardiograms. Another highly talked about program with transformer components is AlphaFold, which demonstrated the ability to predict protein structures at ease, a task that required a decade of intensive analysis. Although transformers are used in modern AI systems, we should not rush to the conclusion that it will be the end of next-gen model developments. It will be a part of the future’s new and emerging range of AI super-tools.
If you liked reading this, you might like our other stories
The Rise In Automation In The Middle East
Can A Machine Learn Morality?