No-Code platforms reduce the repetitive and low-level coding work, replacing it with drag and drop features. But while it tunes typical data science work, are we ready to tackle its underlying effects?
In a survey conducted by PwC, 86 per cent of decision-makers said that AI is becoming a “mainstream technology” at their organisation. A similar report by The AI Journal found that executives mostly anticipate that AI will make their business processes more efficient and help to create new business models and products.
Emerging “no-code” AI development platforms are fueling the adoption further. Designed to abstract the programming typically required to create AI systems, no-code tools enable even non-coders to create machine learning models that can be used to predict inventory demand or extract text from business documents.
Due to the current data science talent shortage, the usage of no-code platforms is now expected to climb up further in the coming years, with Gartner predicting that 65 per cent of app development will be low-code/no-code by 2024.
But there are several potential risks in tuning the way data science can work, sometimes even making it easier to neglect the flaws in the real systems underneath.
The Rise Of No-Code
There are various types of tools that no-code AI development platforms offer to the end-customers, some prominent names are DataRobot, Google AutoML, Microsoft acquired Lobe and Amazon SageMaker. Most provide drag-and-drop dashboards that allow the user to either upload or import data to train, retrain or fine-tune a model or automatically classify and normalise the data for training. Model selection in such tools is primarily automated, as the tool finds the “best” model based on the data and predictions required.
Using a no-code AI platform, a user can upload a data spreadsheet into the interface, make selections from a varied menu, and quickly initiate the model creation process. Depending on its capabilities, specific patterns in text, audio or images can be further spotted.
However, while most platforms imply that customers are responsible for any errors in their models further, tools can cause people to de-emphasise the essential tasks of debugging and auditing the models due to reduced workloads. This phenomenon is known as automation bias, the natural tendency to trust data from automated decision-making systems.
Hazards Of No-Code
A 2018 Microsoft Research study found that too much transparency about a machine learning model makes people, particularly non-experts, become overwhelmed. Another 2020 paper by the University of Michigan in collaboration with Microsoft Research showed that even data science experts at times tend to over-trust and misread overviews of models via charts and data plots, regardless of whether the created visualisations make mathematical sense.
The problem mainly occurs more in cases of no-code computer vision platforms, where the field of AI deals with algorithms trained to “see” and understand patterns from the real world. Computer vision models are already highly susceptible to bias, as even variations in background scenery can affect model accuracy and the varying specifications of camera models. Many experts attribute errors in facial recognition, language and speech recognition systems because of flawed datasets used to develop the models.
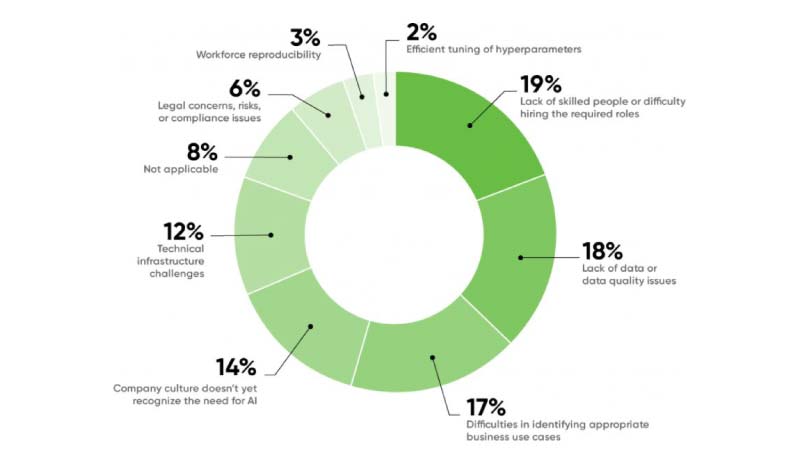
To Be Or Not To Be
Unsurprisingly, vendors have a different perspective on the same. Many feel that anyone creating a model should understand that their predictions will “only be as good as their data.” Today, AI development platforms have a responsibility to educate users about how models make decisions, understand the nature of bias and data modelling to its users. Eliminating bias in model output is best tackled by modifying the training data, ignoring specific inputs so that the model does not learn the unnecessary patterns in the underlying data. Bias reflects “existing human imperfection” that platforms can mitigate but do not have the responsibility to eliminate.
But it has also been seen that training datasets are not solely responsible for model biases that arise. As a 2019 MIT Tech Review lays out, companies might frame the problem that they are trying to solve with AI in a way that does not factor in the potential for fairness or discrimination.
The no-code AI platform they are using might also introduce bias during the data preparation or model selection stages, impacting prediction accuracy. Many companies are now addressing the challenges of no-code platforms and the looming problem of bias in different ways.
DataRobot has recently implemented a “humility” setting that allows its users to educate a model if its predictions are sounding too good to be true than they should be. “Humility” also instructs the model to either alert the user or itself to take corrective measures, like overwriting its current predictions with upper or lower criteria, if its predictions or if the results land outside specific criteria.
For now, the current right path to be followed by vendors could be laying more emphasis on improving education, transparency and accessibility of their tools, while pushing for more descriptive regulatory frameworks.
Businesses using these AI models should be able to easily point to how a model makes its decisions with backing proof from the AI development platform and feel confident in the ethical and legal implications of their use. There is currently a limit to what debiasing tools and techniques can accomplish, and without an awareness of the potential reasons for bias, the chances that such problems crop up in models rigorously increases.
If you liked reading this, you might like our other stories
Garbage In Garbage Out: The Problem Of Data Labelling
Bias in the Training Data Leads to a Biased Algorithm