Technology has reached a point where it can impact not just the way business processes run but also help define what the business is
Technology leaders talked about what enterprises should do to prepare for the future of business. Day 2 at Velocity, the Middle East’s largest in-person conference on data and analytics, in Dubai included speakers such as Zee Hussain – Enterprise Sales Leader for UAE at Qlik, Tomasz Mazur – Head of Customer Acquisition at Chalhoub Group, UAE, James Schlesinger – Senior Product Manager, Web SEO Analytics at Chalhoub Group, UAE, Debbie Botha – Chief Partnership Officer at Women in AI, Keeshan Pillay – Enterprise lead for MEA at Dremio and Edosa Odaro – Author of Making Data Work and Chief Data & Analytics Officer at Tawuniya and many more.
“Today, customers demand greater value,” said Botha, Chief Partnership Officer at Women in AI. She introduced the concept of the cognitive enterprise to prepare for challenges that businesses face today and may encounter in the future. A cognitive enterprise combines disruptive technologies like AI, blockchain, Internet of Things (IoT), 5G, and Edge Computing with innovative processes and data to enhance customer experience and gain a competitive advantage.
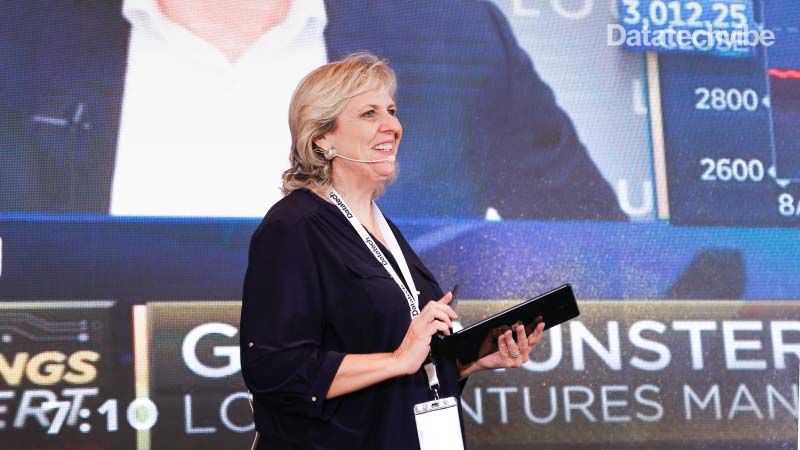
To start, business and tech teams should work together to leverage reinforced and distributed learning in AI while navigating complex business scenarios. It can help teams handle operational efficiency, build better products, and offer more personalised marketing and empathetic customer service. But for this, data needs to flow.
“Within an organisation, we see that each department has its own siloed data repositories. There needs to be a connection within. This can be achieved by upgrading to a Cognitive Enterprise Architecture, a model that continuously learns about connections, behaviour, and interaction,” she said.
Cognitive enterprises draw upon three components:
- an appropriate technology platform
- the corporate culture to deviate from traditional forms of service
- corporate ownership, and deep and meaningful customer engagement
Pillay, Enterprise lead for MEA at Dremio, offers a new data management paradigm. Most enterprises operate both data warehouses and data lakes. For decades, data warehouses have been around, and variations include enterprise data warehouses (EDW), data marts, and operational data stores (ODS). Data warehouses have smartly crafted schemas designed to answer predetermined queries efficiently.
“You don’t need to load the data onto any data warehouse to process and analyse business intelligence requirements. You can directly query the data underlying in your data lakes made of object storage, which will decrease the operational overhead on data pipelining and maintenance,” he says.
Pillay also discussed the advantages of Data Lakehouse, such as eliminating simple ETL through which you can load the data from your existing data lake into your data warehouse by cleansing and transforming it into the destination schema. It also helps reduce data redundancy, simplify data governance, and connect to BI tools.
Sometimes, the best enterprise strategies are loaded with technology, investments, and talent, yet they don’t provide the best results. Why? Odaro, Chief Data & Analytics Officer, Tawuniya, attributes it to four kinds of failures; failure to address fear, failure to embrace counter-intuition, failure to align purpose, and the failure to confront failure itself.
According to the author of Making Data Work, memories feel sharper when things go wrong. The cognitive enterprise will fail without a data-driven culture embraced by all. “If we are looking to change, shift the culture from ‘not working’ to ‘being positive.’ We need to take advantage of the situations where things go wrong as an opportunity to demonstrate what a new world might look like.”
Just like customer expectations have evolved, the methods to judge whether your enterprise is delivering to the point must also evolve. For example, Amazon uses a metric lifecycle that follows a process; define, measure, analyse, improve and control. According to Botha, as a trend, enterprises will move from predicting to prescribing to an autonomous model structure where various teams work towards a unified goal of improving customer experience. This plan needs to be sustainable and scalable for future market dynamics and the changing nature of the business.
As enterprise moves towards a cognitive approach, leaders must be wary of balanced representation. Is it the data that is biased or the teams running the projects? Botha presents a solution that she hopes will soon be a norm in business practice, “Have an inclusive strategy team and use diverse data sets. This is the surest way to reduce bias and noise.”
If you liked reading this, you might like our other stories
Enterprises Don’t Need Big, But Right Data
Is Cognitive Robotic Process Automation A Game-changer?