The Imagen model, trained on many examples, create images that most closely resemble the text
The newest hot trend in artificial intelligence is text-to-image generators, which exhibit skills beyond what the human mind can comprehend. Today’s generator models mimic various styles, including traditional paintings, CGI representations, and even in-depth photos. Technology seems to be developing so quickly that experts predict that such models will soon put human illustrators and stock photographers out of work. Big tech and research firms compete to develop artificial intelligence algorithms that produce high-quality images from simple text prompts.
These have advanced significantly in recent years and typically involve deep neural networks. The most current, Imagen from Google, is immediately followed by DALL-E 2, which OpenAI recently unveiled in April. The Imagen model classifies how photos relate to text descriptions using a neural network that has been trained on a large number of samples. The neural network continuously creates images, modifying them according to what it learned until they most closely resemble the text when a new description is given.
Such learning models show the power of machine learning systems, and models like Imagen remove the need to know how to use specialised editing software like Photoshop to create abstract images. Generator AI systems are assisting the tech industry in realising its vision of an ambient computing future, or the notion that people would one day be able to utilise computers intuitively without needing to be familiar with specific systems or coding.
How does Imagen work?
Imagen uses the massive Transformer language model “T5” to produce an “image embedding,” which is a numerical representation of an image. Initially, the caption is fed through a text encoder, which transforms the textual caption into a numerical representation and extracts the semantic data from the text. After starting with noise and gradually changing it into an output image, an image-generation model produces an image. The text encoding is given to the picture-generation model as input to help with this process since it informs the model exactly what the caption implies so it can produce a similar image. The generated image is then sent into a super-resolution model, which increases the resolution of the image.
The resulting image, 1024 x 1024 pixels, graphically conveys the meaning of our caption. Such AI scaling enhances the original image with new features, resulting in a target resolution with excellent sharpness. Additionally, Google created the Economical U-Net deep learning architecture for Imagen, which is “simpler, converges faster, and is more memory efficient” than earlier U-Net implementations.
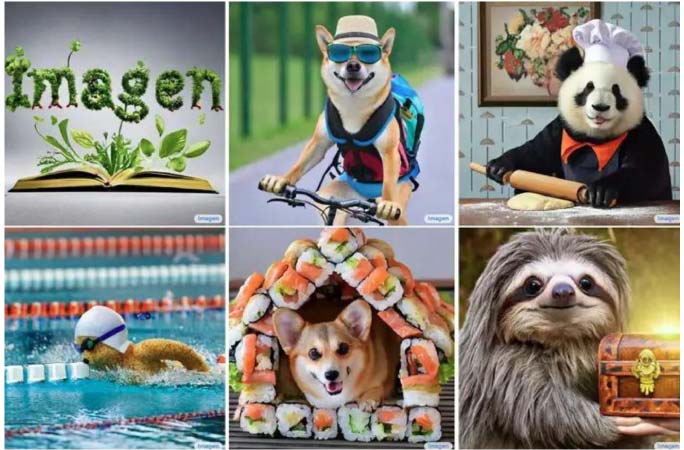
Beating contemporaries
The Brain Team at Google Research tested the model and compared it to existing text-to-image tools like DALL.E 2, CLIP, and VQ-GAN+CLIP in a study titled Photorealistic Text-to-Image Diffusion Models with Deep Language Understanding. In terms of photorealism, Imagen was thought to be superior to its rivals. Imagen, unlike many other models, was pre-trained entirely on text data and outperformed DALL-E 2 on the COCO benchmark. Imagen topped DALL-E 2, the prior best-performing model, with a zero-shot FID score of 7.27.
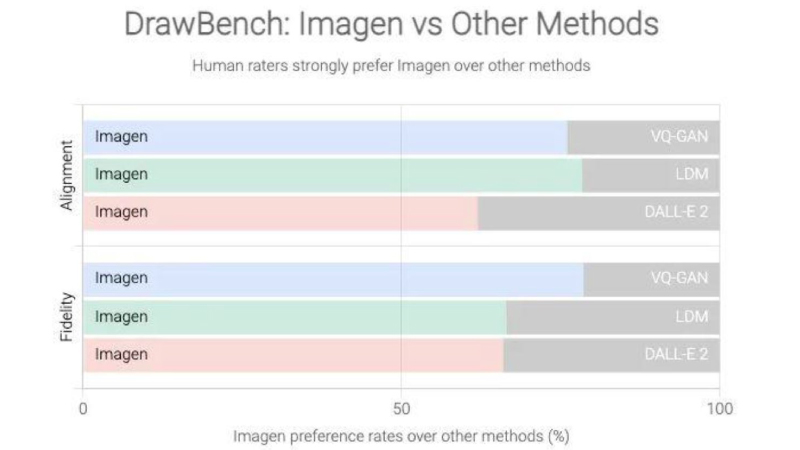
Although text-to-image generators produce amazing graphics, some researchers have questioned whether the best outcomes were handpicked to represent the systems. The fact that corporations have so far refused to offer public demos that would allow researchers and others to try them out and are only available to select beta testers presents a challenge in adequately evaluating these AI-generated creations. One of the factors is a concern that AI might be used to produce false images or produce adverse outcomes.
Are we there yet?
Often, images generated by text-to-image models look unfinished, smeared or blurry, problems that were seen with pictures generated by OpenAI’s DALL-E program. In its investigation, Google found that these systems also contain social biases and that their output can occasionally be poisonous in a racist, sexist, or another creative way. The vast scale data requirements of text-to-image models have forced researchers to rely extensively on large, mostly uncurated, web-scraped datasets, according to the Google researchers’ summary of the issue in their study. Audits of these datasets have shown that they occasionally reflect social prejudices, oppressive ideologies, and detrimental linkages with marginalised identity groups.
Keeping this in mind, Imagen filters specific text inputs to prevent the model from producing offensive, violent, or racist imagery. The history of artificial intelligence tells us that such text-to-image models will indeed become mainstream at some time in the future, with all the unsettling implications that greater access implies. These safeguards, in a sense, do restrict potentially destructive applications of this technology. As of now, Google concluded that Imagen “is not fit for public usage at this time,” and the company says it aims to create a new method of measuring “social and cultural bias in future work” and testing fresh iterations.
Despite this, the friendly competition between the large corporations will probably result in the technology advancing quickly because tools created by one group can be included in another model. The sheer amount of computational power needed to train these models on massive amounts of data tends to restrict work around them to significant players. However, smaller teams may eventually offer similar technologies.
If you liked reading this, you might like our other stories
Is DALL-E 2 The Next Big Revolution In AI Research?
AI Predicts Race From X-Ray Images