Poor data quality costs organisations an average of $12.9 million, reports Gartner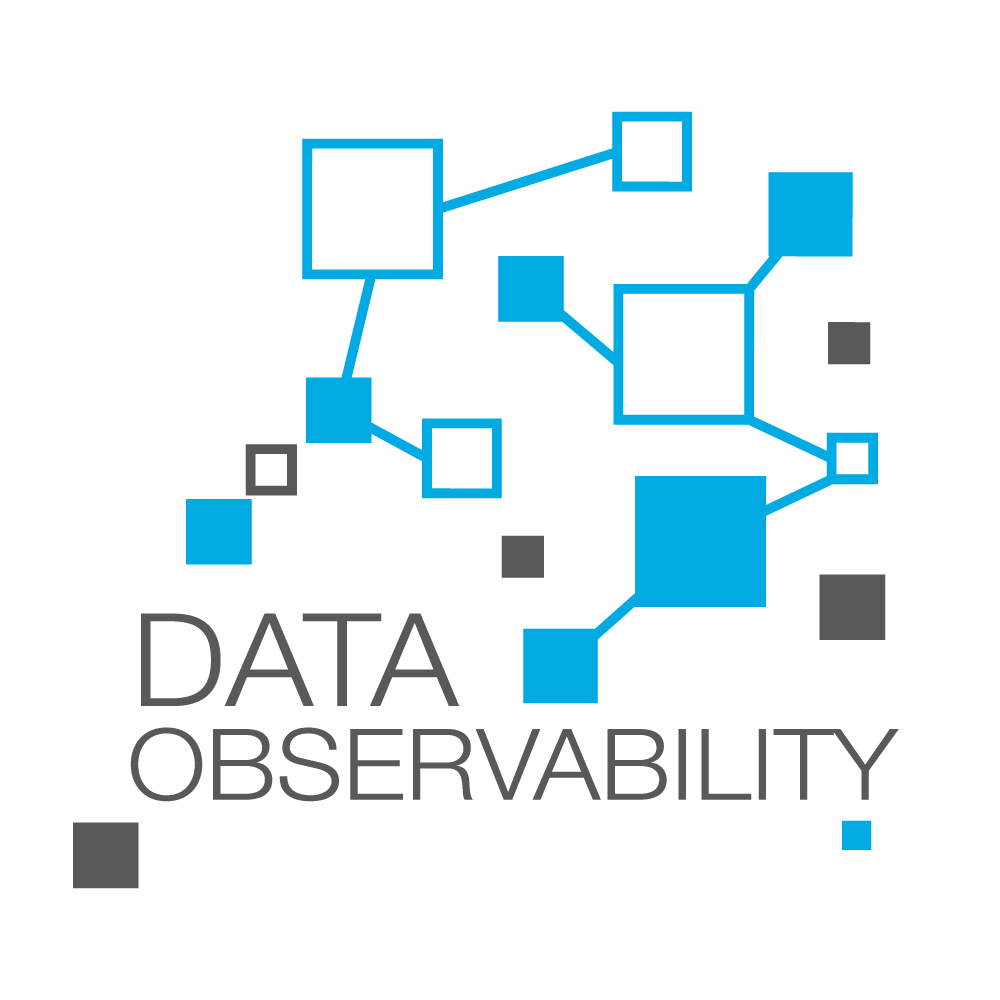
The value of any organisation can be measured by its data quality. It’s the capability of the data to serve an intended purpose, an essential aspect of a data-driven organisation. Higher quality data provides timely and accurate information to manage accountability and services, also aiding in ensuring and prioritising the best use of resources. Maintaining high-quality data leads to appropriate insights for decision-makers and accurate analytics. With big data and AI growth, organisations collect vast amounts of data, and managing its quality becomes more critical every day.
But maintaining high-quality data health remains a challenge for many businesses, and poor quality data can result in significant brand damage, customer losses, sales deficits, and high employee turnover. Poor data quality costs organisations $12.9 million, reports Gartner.
“Data has also become much more operational,” said Rohit Choudhary, CEO and Co-founder of Acceldata, a provider of data observability solutions. “In the past, data was primarily used to populate monthly or quarterly reports to give people a snapshot of their businesses at a given point in time. Now, data powers a variety of real-time use cases that are core to business operations, and that is putting extra pressure on data teams to ensure the quality of data.”
The importance of data quality
According to a Forrester report, almost 30 per cent of analysts spend 40 per cent of their time validating and vetting their data before its utilisation for strategic decision-making and algorithmic implementations. These statistics indicate the scale of the data quality problem. Bad data quality can also overlook important events, misdiagnose problems, or prescribe the wrong solution to a pressing issue.
But why do these data quality challenges occur? The main reasons include complex hybrid data stacks, gaps in skill knowledge and shortages and insufficient testing time. Data mismanagement often leads to dubious internal practices. In a survey by Validity, 75 per cent of data practitioners admitted that they usually (33 per cent) or sometimes (42 per cent) fabricate data to tell the story they want decision-makers to hear.
“When something breaks or goes wrong, data engineers don’t have the context to understand the issue,” said Choudhary. “It creates a compounding effect that places even more pressure on data teams to effectively monitor everything. Data observability gives you end-to-end visibility into the health of your data and pipelines, and gives you context to understand why things break or fail.”
Acceldata’s multidimensional data observability platform improves the reliability, productivity and cost of data management.
Acceldata is:
- Comprehensive: Monitors the usual data quality concerns as well as many other risks, including reconciling data in motion, schema drift, and data trends and anomalies to provide comprehensive data reliability
- Automated: Leverages machine learning and an easy-to-master, user-friendly UI to make quick work of managing data reliability across large and diverse data environments
- Scalable: Supports on-premises, hybrid and cloud architectures to ensure data moves at the speed of modern business
Data quality and reliability management
Data is considered high quality if it meets the intended purpose of its use, even though there are no widely agreed-upon criteria. High-quality data is defined by several characteristics: correctness, completeness, relevance, timeliness, and consistency. Data quality management lays the groundwork for all of a company’s initiatives. It blends data, technology, and organisational culture to offer relevant and reliable outcomes.
- Monitoring and purifying data is the first step in enhancing data quality. This validates data against matching descriptions and uncovers relationships by comparing it to established statistical metrics. This also verifies the data’s uniqueness and assesses its reusability.
- The second is central metadata management. Multiple employees collect and sanitise data regularly, and they may be located in different countries or offices. As a result, one needs clear regulations on obtaining and maintaining data because employees from other departments may misunderstand specific data phrases and concepts. The solution to this challenge is centralised metadata management, which reduces conflicting interpretations and aids in establishing company standards.
- Data is frequently acquired from various sources and may include numerous spelling alternatives. As a result, segmentation, scoring, smart lists, and various other functions are affected. As a result, a unified approach is required to enter a data point, and data normalisation provides this solution. The purpose of this method is to eliminate data redundancy. Its benefits include improved consistency and more straightforward object-to-data mapping.
- Determine how business processes, key performance indicators (KPIs), and data assets are linked. Make a list of the organisation’s current data quality challenges and how they are affecting revenue and other business KPIs. Data and analytics executives can start constructing a targeted data quality improvement programme that clearly outlines the scope, a list of stakeholders, and a high-level investment strategy by establishing a connection between data as an asset and the required improvements. Data analytics leaders must establish data quality standards that can be applied across the organisation’s business units. Different stakeholders in an enterprise are seen to have different levels of business sensitivity, culture and maturity, hence the manner and speed with which requirements of data quality enablements are met may certainly differ.
- A data quality dashboard provides all stakeholders with a comprehensive snapshot of data quality, including historical data, to identify trends and patterns that can aid in the design of future process improvements. It can be used to track the performance of data that is critical to business processes over time and can be tailored to a company’s specific needs. Incorporating new data practices can positively impact operational business processes, further reflected in data quality dashboards.
- The impact of the improvement programme must be assessed, and leaders must disseminate the outcomes on a regular basis. A 10 per cent improvement in customer data quality can be linked to a 5 per cent improvement in customer responsiveness, as they can be serviced quicker and better by customer care executives with readily available good-quality and trusted data.
Quality data will lead to more efficient data usage while reducing costs across the organisation. In an era of data overload, standalone data quality tools need to be further combined with solutions that offer real-time processing across all lines of business and do not require data engineer-level knowledge to use. With much data already digitised, it is essential to find cost-effective solutions for data onboarding utilising third-party data sources for publicly available data.
If you liked reading this, you might like our other stories
Data Reliability Engineering Is The Partner BFSI Firms Need
The Digital Certificate Conundrum