AI-driven XDR extends continuous threat detection and monitoring and automated response beyond endpoints to provide deeply contextual correlations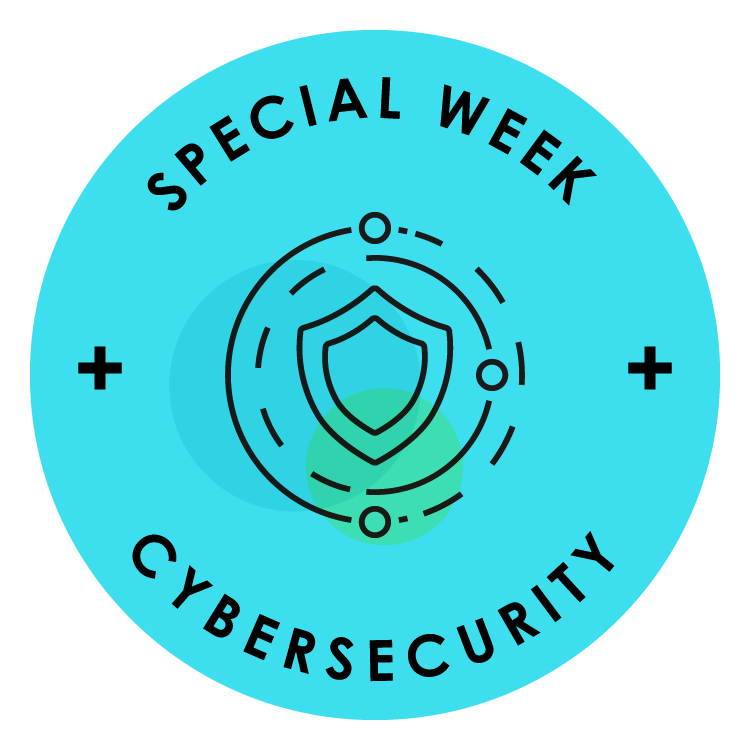
Many security professionals expect an uptick in AI-assisted attacks shortly. According to AIMultiple, 90 per cent of InfoSec personnel in the US and Japan said they’re anticipating an increase in these automated attack campaigns, due in no small part to the public availability of AI research, which attackers are exploiting for their malicious purposes.
How attackers are abusing AI
The findings from AIMultiple’s survey raise an important question: how might digital attackers use AI for their nefarious ends? Let’s look at two applications. First, attackers can use AI to increase the effectiveness of their operations. Time and time again, there are examples of AI helping threat actors create more convincing videos and emails, leading to more clicks by targets.
Attackers can also use AI to help evade detection and bypass traditional security solutions. AIMultiple specifically noted that deepfakes in attacks could allow threat actors to evade biometric security solutions, fly under the radar of social network protections, and ultimately gain access to a target’s corporate data.
Second, malicious actors can use AI to identify potential opportunities for an attack in the reconnaissance phase. Because of the amount of data intelligent systems can collect and analyse autonomously, AI-powered tools provide attackers with a means for identifying vulnerabilities in a target’s networks, devices, and applications at scale – sometimes before security pros know about them.
In the absence of real-time monitoring solutions, they might then be able to move laterally across the network, exfiltrate sensitive information, and increase the overall damage from the attack beyond what they could do without AI capabilities. AI can allow attackers to automate these processes in target selection and customising the attack sequence based on the specifics of a target’s network makeup by determining items like operating systems in use, detecting sandboxing and other defensive tactics, and determining the right payload to deliver.
Fighting AI with AI
In response to these nefarious use cases for attackers, organisations can fight AI abuse with AI-driven solutions to protect their systems and data from automated attacks. Still, traditional security solutions that rely on manual triage and investigation won’t cut it.
Take Security Information and Event Management (SIEM) as an example. These tools can help organisations to centralise threat information across their environments. However, SIEMs tend to generate large volumes of alerts and false positives that waste security professionals’ time and contribute to alert fatigue.
It’s one thing if SIEMs could help that personnel address all those alerts, but they don’t. They point out potential threats that will require human investigation to find correlations between the alerts generated to determine if there is an actual threat and then mount a manual response.
Consequently, security teams need to rely on manual processes to figure out what’s happening across their environments, where attackers are moving at machine speed by leveraging automation in the early stages of the attack, which puts defenders at a great disadvantage.
Endpoint Detection and Response (EDR) solutions are essential to defending endpoints that make up a significant portion of an organisation’s attack surface, but they similarly suffer from shortcomings. While EDR is significantly better at picking up on advanced threats than traditional antivirus and antimalware solutions, many attackers have evolved their campaigns beyond just targeting the endpoint or have designed their operations to minimise endpoint activity, rendering EDR ineffective. EDR cannot also correlate endpoint detections with telemetry from non-endpoint assets like application suites, user personas, and cloud workloads.
AI-powered XDR
Fortunately, organisations don’t need to settle for incomplete solutions like SIEMs and EDR. They can choose to go with an AI-driven Extended Detection and Response (XDR) solution. AI-driven XDR extends continuous threat detection and monitoring and automated response beyond endpoints to provide deeply contextual correlations with telemetry from applications, identity and access tools, containerised cloud workloads, and more.
AI-driven XDR also ingests threat intelligence streams to allow organisations to defend against known attacks and uses AI and machine learning (ML) to automatically correlate telemetry from across these different assets to deliver the complete attack story in real-time. This functionality frees security analysts from needing to triage every generated alert, enabling them to address actual threats faster.
Many organisations are turning to tools powered by artificial intelligence (AI) and machine learning (ML) to allow their teams to automate triage, investigation, and remediation efforts at scale. Over half (52 per cent) of executives at US companies told PwC that they had accelerated their AI/ML adoption plans, and even more (86 per cent) said that AI/ML would be a “mainstream technology” in their environments by the end of 2021.
What’s more, AI/ML can enable security teams to cut through the noise produced by a constant flood of threat alerts, allowing security professionals to spend less time sifting through alerts and chasing false positives and more time working to improve the organisation’s overall security posture.
AI/ML technologies excel at analysing large-scale data sets with high accuracy to identify suspicious events at a speed and volume that manual human analysis can never match. The advantage here is in automating the detection of events that previously required human analysis and relieving security teams of the tedious task of sorting the signal from the noise.
AI-driven XDR also leverages behavioural analytics and Indicators of Behavior (IOBs) to provide a more in-depth perspective on how attackers conduct their campaigns. This operation-centric approach is superior at detecting earlier attacks, especially highly targeted attacks that employ tools and tactics that evade traditional endpoint security software.
Finding one component of an attack via chains of potentially malicious behaviour allows defenders to see the entire operation from the root cause across every impacted user, device, and application. This is where AI-driven XDR is essential to automatically correlate data at millions of events per second versus analysts manually querying data to validate individual alerts over several hours or even days.
Such visibility enables security teams to respond to an event before it becomes a major security issue and introduce measures designed to increase the burden on attackers.
The application of AI is not a silver bullet, and for the foreseeable future, there will undoubtedly need to be a blend of humans and AI-driven solutions working together. Nonetheless, AI will enhance the efficiency of every member of the security team and amplify the efficacy of the entire security stack.
If you liked reading this, you might like our other stories
Adding AI to Supply ChAIn
Three Reasons Why XDR Should Drive Your IT Strategy