Recently, every big tech conglomerate has announced its own Multimodal System, termed as the future of AI. But what is this emerging domain all about? Does it really have the revolutionary potential to do what it promises?
In June, Google introduced MUM, a model trained on a dataset consisting of many documents from the web that can transfer knowledge between languages. MUM does not need to be explicitly taught how to complete tasks and can answer questions in 75 different languages. The trend of creating an AI system that is enabled with complete human-level intelligence and perception is now evolving into a competitive space between tech giants. Humans can understand the meaning of text, videos, audio, and images holistically with context. Nevertheless, even the best AI systems seem to struggle in this area. While systems somewhat capable of making these multimodal inferences remain beyond reach, there has been much progress.
Multimodal Systems and Its Potential
A Multimodal system is an AI architecture, where computer vision and natural language processing models are trained together on multiple datasets to learn a combined embedding occupied by variables representing specific features of the images, text and other media. Multimodal systems can be used best to predict image objects from text descriptions or a body of literature, specifically in the subfield of visual question answering, also known as VQA, a computer vision problem where a system is given a text-based question about an image and must infer the answer.
Not long ago, multimodal learning seemed to be in its infancy and faced formidable challenges, but breakthroughs have been made. Earlier this year, OpenAI released two advanced multimodal models that the research labs claim is “a step forward towards systems with a deeper understanding of the world”, named DALL-E and CLIP. Artist Salvador Dalí inspires DALL-E, and the model is trained to generate images from simple text descriptions. On the other hand, CLIP, i.e. Contrastive Language-Image Pretraining, is trained to correlate visuals with language, drawing on sample photos paired with captions scraped from the public web.
A more recent project by Google named Video-Audio-Text Transformer or VATT attempts to build a competent multimodal model by training the system with datasets that comprise video transcripts, videos, audio, and photos. VATT can also make predictions for multiple modalities and datasets directly from raw signals, successfully captioning the events in videos and categorising audio clips and visually recognising objects in images.
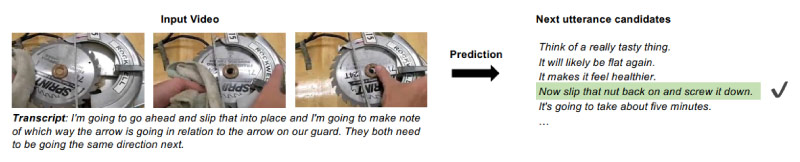
Other recognised AI labs have also joined the race. Researchers at Meta came up with FLAVA and Microsoft Research Asia, and Peking University researchers developed NUWA, both of which can recognise real-world modalities. However, there is still just one problem. Multimodal systems can notoriously pick up on biases in datasets.
The Looming Danger of Bias
Like other models, multimodal models too are susceptible to bias, which often arises from the datasets used to train the models. The required diversity of questions and concepts for tasks such as the VQA and the dearth of high-quality data often prevent models from learning to reason, leading the models to make guesses by relying on the provided dataset and statistics. OpenAI explored the presence of biases in multimodal neurons, the core components that make up multimodal models, including a “terrorism” neuron that responds to images of words like “attack” and “horror”.
A benchmark test developed by a group of scientists at Orange Labs and Institut National des Sciences Appliquées de Lyon claims that the standard metric for measuring VQA model accuracy is often misleading. They offer an alternative GQA-OOD, which evaluates performance on questions whose answers cannot be inferred without reasoning. While conducting a study involving 7 VQA models and three bias-reduction techniques, the researchers found that these models failed to address questions involving infrequent concepts, which suggested further work to be done in this area.
The current solution seems to involve more extensive, more comprehensive training datasets. With better datasets, new training techniques might also help boost multimodal system performance. According to the researchers, pretraining tasks and a larger dataset with million image-text pairs might help VQA models learn with better-aligned representation between words and objects.
Multimodal Systems For Real-World Scenarios
While some work remains to be addressed and seems firmly in the research phases, companies including Google and Meta are actively commercialising multimodal models to improve their products and services. Google says it will use MUM to power a new feature in Google Lens, for the company’s own image recognition technology, that finds objects like apparel based on photos and high-level descriptions. Google also claims that MUM has helped its engineers identify more than 800 COVID-19 name variations in over 50 languages.
Meanwhile, Meta is reportedly using its multimodal models to recognise whether certain memes are violating its terms of service and fairness. The company has recently built and deployed a Few-Shot Learner (FSL) system that can adapt itself to take action on evolving types of potentially harmful content in more than 100 languages. Meta claims that when deployed on Facebook, FSL has helped identify specific content that shares misleading information in a way that would discourage the reader of COVID-19 vaccinations or that comes close to inciting violence.
Although certain developments for multimodal systems have been quite fruitful in terms of actually yielding machine learning models that let us now perform more sophisticated NLP and computer vision tasks than before, it has its own set of challenges. Multimodal systems hold immense promise once it overcomes the technical challenges. Will it be the future of automated AI? Let us wait and see.
By combining information from different modalities into one universal architecture currently seems promising not only because it is equivalent to how humans make sense of the world but also because it might just lead to generating better sample efficiency and richer representations.
If you liked reading this, you might like our other stories
Let Augmented Analytics Do Your Heavy Lifting
5 Native Advertising Rules Every Marketer Should Know