Cognitive AI competencies will help unlock the capabilities for systems that are more explainable and capable of applying reasoning closer to humans
With recent technological breakthroughs, support systems today use better data and algorithms to comprehensively analyse a massive amount of information. Tasks requiring human perceptual and cognitive skills can now be easily automated. A product of the current artificial intelligence research, cognitive technologies are constantly evolving. Tech giants are now turning their focus as cognitive AI’s prowess has exponentially increased in recent years, with developments in computer vision, natural language processing, speech recognition, and robotics, among other areas.
Cognitive technologies aid in extending the power of information technology, as they have the potential to enable organisations to break prevailing tradeoffs between speed, cost, and quality.
Next-gen potential
The demand for a broader set of AI use cases calls for a substantially new approach that integrates information and knowledge in deep learning-based systems to handle real-life situations. Deep learning is highly susceptible to variations in data and can produce implausible classifications, which could be addressed when improving robustness and extensibility. For the most part, neural networks cannot correctly provide abilities such as cognition, reasoning, and explainability. Deep learning currently lacks the cognitive mechanisms that can address tasks fundamental to human intelligence or missing competencies such as abstraction, context, causality, or intelligible reasoning.
Over the next five years, it is expected that the impact of cognitive technologies on organisations will grow substantially. An AI that can make reliable decisions in unforeseen circumstances will eventually be trusted and become significant in areas such as robotics, autonomous transportation, and control points of logistics, industrial, and financial systems. An increased level of human-machine collaboration is expected in the future, as cognitive AI represents active and persistent agents that can communicate and collaborate with people, as it serves and learns from them.
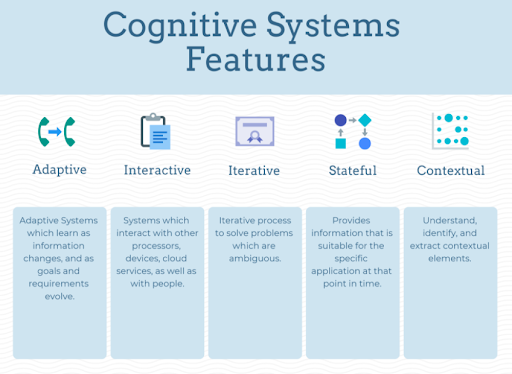
As mapping functions become significantly more elaborate and knowledgeable, with compute processing becoming faster and cheaper, cognitive AI will enable an additional level of sophisticated capabilities. We can see promising early efforts to integrate structured knowledge with deep learning to build more generative and dynamic systems by formulating traditional tasks such as commonsense question-answering as inference over dynamically generated knowledge graphs.
The emerging focus and research on symbolic-based approaches founded on structured knowledge indicate that a new set of cognitive AI competencies will help unlock the capabilities needed for systems that are more explainable and apply a level of autonomous reasoning closer to humans than the current deep learning-based systems. The next level of machine intelligence will require reasoning over deep knowledge structures, including facts and deep networks of declarative (know-that), causal (know-why), conditional/contextual (know-when), relational (know-with), and other types of models. The capture and use of deep knowledge can address fundamental challenges of AI, such as the difficulties presented by the explosion in Deep learning model size and gaps in model robustness, extensibility, and scaling.
Cognitive AI use cases
- AI-enabled cybersecurity: Cyber-attack prevention software can use data security encryption and enhanced situational awareness, powered by AI. This provides document, data, and network locking through smart distributed data, secured by an AI key.
- Content AI: Solutions powered by cognitive intelligence continuously learn and reason, simultaneously integrating location, time of day, user habits, semantic intensity, intent, sentiment, social media, contextual awareness, and other personal attributes.
- Cognitive analytics in healthcare: The technology implements human-like reasoning software functions that can perform deductive, inductive, and abductive analysis for several life sciences applications.
- Intent-based NLP: Cognitive intelligence can help businesses become more analytical in their management and decision-making approach. This works as the next step from machine learning, and the future applications of AI will incline toward using this for performing logical reasoning and analysis.
Understanding how to derive maximum benefit from cognitive technologies requires a deeper analysis of a particular organisation’s process, data, talent model, and market. It is true that the use of cognitive technologies is not viable everywhere, and neither is it valuable everywhere. But one of the most vital uses of cognitive technologies would be creating value in AI model development. For most applications, cognitive technologies will restructure work and make it more efficient.
If you liked reading this, you might like our other stories
Is Cognitive Robotic Process Automation A Game-changer?
Can Micro-drilling Prepare Your Crisis Response Team?