The new model provides an outlook of how generative deep learning models might finally unlock new creative applications for everyone to use
Generative models are a trending area of research, receiving attention since generative adversarial networks (GAN) were introduced in 2014. In recent years, the field has seen impactful improvements, and generative models have been used for a wide spectrum of tasks, including creating artificial faces, deepfakes, synthesised voices, and more. Known for presenting state-of-the-art models through exhaustive research, OpenAI’s DALL-E Artificial Intelligence (AI) model has again created a buzz around the research space.
The recently announced DALL-E 2 that can generate stunning images from mere text descriptions shows how far the AI research community has harnessed the power of deep learning and addressed some of its limits. The new model provides an outlook of how generative deep learning models might finally unlock new creative applications, at the same time reminding us of the obstacles that remain in AI research and disputes that need to be resolved.
The improved prowess
DALL-E 2 presents an improved version, learning from the success of its predecessor. The prior version was known for the quality and resolution of output images using advanced deep learning techniques. A generative model presents complex outputs instead of traditional prediction or classification tasks from the input data. If you provide DALL-E 2 with a text description, it can generate multiple images that fit the description. However, what sets the latest development apart from other generative models is the capability to maintain a semantic consistency amongst the images it creates. The model retains its consistency in drawing, delivering a photorealistic style output from image inputs. The model can also generate other forms of the same input image, maintaining and reproducing the relationships between the elements in an image.
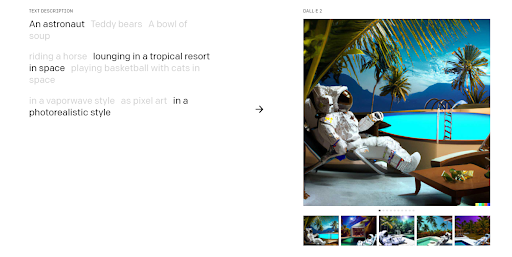
How it works
DALL-E 2 is built upon CLIP and diffusion models, two of the most advanced deep learning techniques created in recent years. At its heart, it shares a similar concept as other present deep neural networks: representation learning. Considering an image classification model, the neural network first transforms pixel colours into a set of numbers that represent its features. This vector is sometimes also known as the “embedding” of the input. The features are then mapped to the output layer, which contains a probability score for each image class that the model is supposed to detect. The neural network then tries to learn the best feature representations that discriminate between the classes during training.
Ideally, a machine learning model should learn latent features that remain consistent across different lighting conditions, angles, and background environments. But as often seen, deep learning models tend to learn false representations. For example, a neural model might think that green pixels are an important feature of “sheeps” because all the images of sheep it saw during training contained much grass. Another model trained on pictures of bats taken during the night might consider darkness as a feature of all bat pictures and misclassify with the images of bats taken during the day. Other models might also become sensitive to objects being centred in the image and placed in front of a specific type of background.
Here’s DALL-E 2’s output when you ask for a flight attendant:
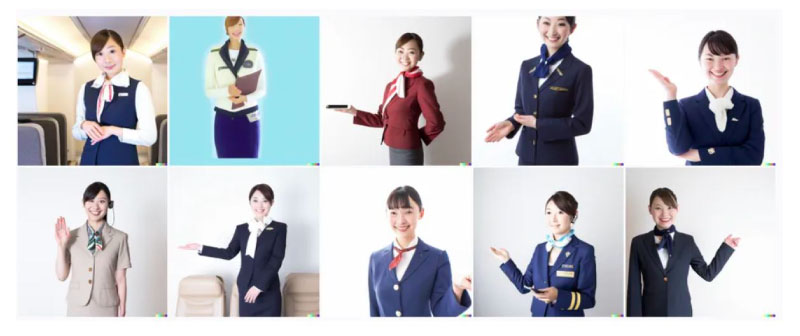
Are they yet biased?
OpenAI is well aware that the released version of DALL-E 2 generates results that exhibit gender and racial bias. The researchers are still trying to resolve bias and fairness problems but could not rule out problems effectively because different solutions result in different trade-offs. OpenAI is far from being the only artificial intelligence company dealing with bias and trade-off issues; instead, it’s a challenge to ponder upon for the entire AI community. Before DALL-E 2 was released, OpenAI invited 23 external researchers to the “red team,” i.e., to find as many flaws and vulnerabilities as possible to improve the system. One of the leading suggestions that came through the process was to limit the initial release to only trusted users, and OpenAI adopted this suggestion. For now, only 400 people, a mix of OpenAI’s employees and board members plus hand-picked academics and creatives, get to use DALL-E 2, that too only for non-commercial purposes.
OpenAI has been trying to find the right balance between scientific research and product development. Many analysts suggest multiple applications for DALL-E 2, such as creating graphics for articles and performing basic edits on images. DALL-E 2 will enable more people to express their creativity without the need for special skills with tools. The applications of DALL-E will surface as more users tinker with it. Suppose OpenAI releases a paid API service just as it did for GPT-3. In that case, many users will be able to develop apps or integrate the technology with DALL-E 2 into existing applications.
The current advances in AI are taking us towards a world in which good ideas are the limit for our potential and not specific skills. Building a concrete business model around the potential of DALL-E 2 will have its unique challenges. Much of it will depend on the costs of training and running DALL-E 2. As the exclusive licence holder for GPT-3’s technology, Microsoft might be the primary winner of any innovation built on DALL-E 2, doing it faster and cheaper. Like GPT-3, DALL-E 2 reminds us that as the AI community continues to gravitate toward creating more extensive neural networks trained on ever-larger training datasets, tech giants with the financial and technical resources needed for AI research may still remain in power.
If you liked reading this, you might like our other stories
You GAN Do Whatever You Want
Neural Networks: The Deeper, The Better?