More organisations are turning to synthetic data to feed their machine learning algorithms. It’s an inexpensive alternative to real-world data
One certainty of deep learning is that larger, more diverse datasets always lead to more robust models. With metaverse, smart automotive, and the rise of data-centrism, there is a demand for high-quality, targeted data. AI pioneer Andrew Ng is rallying support for a benchmark on data quality, which many claim represent 80 per cent of the work in AI.
Ostensibly, machine learning (ML) needs lots of high-quality data to work well, but according to the findings of a recent Datagen survey, a stunning 99 per cent of respondents reported having had an ML project cancelled due to insufficient training data, and all reported experiencing project delays as a result of inadequate training data.
Moreover, for organisations having in-house data teams to collect, compile, and annotate their data, there are chances of biases, inadequate edge-case performance, and privacy violations slipping into the AI model.
Enter synthetic data. It is a promising way to increase dataset size and diversity and allow organisations to build stronger models and get around the challenges of obtaining and using high-quality data from the real world. It can be even better for training an AI model than data based on actual objects, events or people, a research states.
Another report calls the use of synthetic data “one of the most promising general techniques on the rise in modern deep learning, especially computer vision” that relies on unstructured data like images and video.
Synthetic data, which is computer-generated, photo-realistic image data, offers solutions to almost every item on the list of mission-critical problems computer vision teams currently face, gaining widespread acceptance among computer vision experts.
Rendered.ai, which has a complete stack for synthetic data, including a developer environment, a content management system, scenario building, and compute orchestration, improved object-detection performance outcomes through synthetic data for Orbital Insight. Rendered.ai helped them modify synthetic images, so the trained AI model can generalise to real images. They also used a large set of synthetic images and a small set of real examples efficiently to train a model jointly.
Rendered.ai has proven that real-world datasets based on simulation of a 3D environment can be used to both increases AI performance and reduce the amount of data used to train AI.
Wide-ranging benefits
GANs (Generative Adversarial Networks) is a common method used to generate synthetic data. Researchers from Ford combine gaming engines and GANs to create synthetic data for AI training. They deploy this sim-to-real pipeline on HPC clusters and quickly generate large amounts of data. This sim-to-real method reduces the time and costs it usually takes to collect and annotate real-world data.
In healthcare, medical imaging uses synthetic data to train AI models while protecting patient privacy. Curai, for example, trained a diagnostic model on 400,000 simulated medical cases.
Even American Express studied ways to use GANs to create synthetic data, refining its AI models that detect fraud.
Meanwhile, retail companies enable smart stores where customers grab what they need and go without waiting in a checkout line, using synthetic datasets. Caper, for example, used synthetic images of store items that capture different angles and trained its algorithm with it. Its shopping carts have 99 per cent recognition accuracy, the company claims.
Synthetic data offers many benefits, allowing the quicker, less resource-intensive generation of high-quality, targeted datasets for ML model training. As a result, teams can adopt a data-centric approach to ML development, iterating quickly with robust and refined datasets.
According to the Datagen survey, respondents showed near-uniform support for reduced time-to-production (40 per cent), elimination of privacy concerns (46 per cent), reduced bias (46 per cent), fewer annotation and labelling errors (53 per cent), and improvements in predictive modelling (56 per cent).
The idea is to generate realistic data and include everything that the AI model needs to learn and comes pre-annotated, thereby lowering cost.
Synthetic data adoption is growing – of those not currently using synthetic data, over 38 per cent plan on using synthetic data this year, according to Datagen.
Last year, Gartner predicted by 2030, most of the data used in AI will be artificially generated by rules, statistical models, simulations, or other techniques. “The fact is you won’t be able to build high-quality, high-value AI models without synthetic data,” the report said.
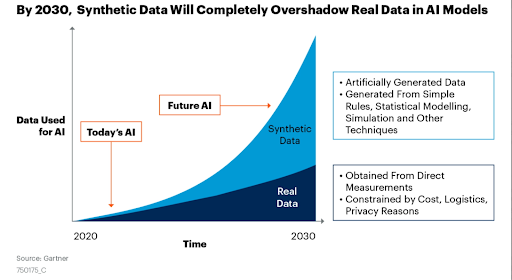
With instances of AI bias aplenty – the most talked about, however, are Google’s hate speech detection algorithm discriminated against people of colour and Amazon’s HR algorithms were fed with mostly male employee data for ten years, synthetic data represents a step forward to solve the problem.
Suppose the dataset is not diverse or large enough. In that case, synthetic data, generated partially or completely artificially rather than being extracted from the real world, can fill in the holes and form an unbiased dataset. Even if an organisation’s sample size is large, there’s a big chance of certain people being excluded or unequally represented; synthetic data can resolve the problem.
Also, gathering actual data requires measuring, interviewing, a large sample size, and, in any case, plenty of work. AI-generated synthetic data is cheap and only requires ML techniques.
Let’s take facial recognition, for example. Feeding an AI algorithm with unequal face data creates bias. If the training sets aren’t that diverse, synthetic data can create full-spectrum training sets that reflect a richer portrait of people. Generating enough synthetic AI data representing artificial faces of people of different races and ethnicities and feeding it to the AI algorithm, the error rate will be the same for all races.
Additionally, generating synthetic faces helps to avoid any privacy issues. When AI and data privacy concerns many Internet users, generating synthetic data (of faces, in this case) is a safer option.
Interestingly, synthetic data also effectively mitigates edge-case failures, as one can make fast, inexpensive, and targeted additions to one’s dataset with each iteration. For example, suppose a CV team discovers that their autonomous vehicle is frequently mistaking sandstorms for rain, and so needlessly reducing speed and engaging traction control in response. In that case, rather than going to Dubai, or any other Middle Eastern city, to check the wind condition, they can simply generate synthetic, computer-generated images with sandstorm conditions in place.
Also, using synthetic data would spare the team the additional time of preparation and annotation that manually collected data require.
Though the space is only a few years old, more than 50 companies already provide synthetic data, each focusing on a particular vertical market or technique.
The benefits of synthetic data leave little doubt that its use will increase in the coming years as third-party synthetic data platforms and providers scale to meet this demand.
If you liked reading this, you might like our other stories
Companies To Watch
The 6 Rs Of Cloud Migration You Cannot Ignore