Using A/B testing with data science, businesses can discover options that would offer better returns and get rid of unwanted processes
Data supports the best business decisions, and tech companies have figured out that data is their product. Whether one sells a service, a product, or content, the end goal is to create value for the customer base. Every interaction with the product comprises a measurable amount of value. However, the best data-driven companies do not just passively store and analyse their data, they actively generate actionable data by running several experiments. The secret to churning more value from data is testing, and for data-driven businesses today, implementing well-executed, consistent A/B testing is necessary.
The Value Of Testing
A/B testing, at its core, involves analysis through different user experiences to different users to measure the impact of those differences. A/B testing is a tried method to take UX concepts for a test drive in terms of a digital universe. These controlled experiments rigorously validate the most robust hypotheses before turning an idea into reality. Nevertheless, A/B testing can cause mishaps that hurt rather than help a business when it is not appropriately done.
The most visible benefit from a test like this is the ability to improve products and increase our revenue through data-driven decision making. But there are also indirect benefits of testing, ones that manifest themselves once testing becomes ingrained in company culture. A/B testing is an essential way to get to the bottom of a decision without relying on anyone’s gut instinct.
No two marketing campaigns will ever offer similar returns; one will always somehow be better than the other. With the help of A/B testing using data science, businesses discover options that would offer better returns and eliminate unwanted processes that offer lower returns and spend the money where it pays more.
AB testing helps increase profits by improving conversions and allowing the business to reach more people. About 60 per cent of businesses believe it helps improve conversion.
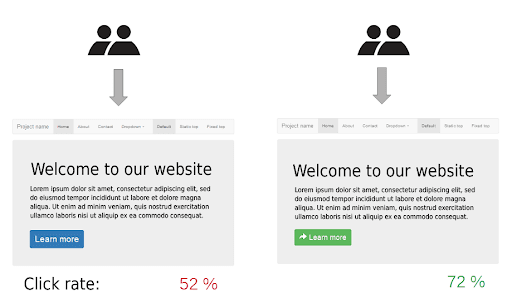
Best Practices Aiding Data-Driven Decisions
One of the best ways to determine if A/B testing is successful is to create a holdout group, a small percentage of people who do not interact. For every new feature added to the product, you need to preserve a group of users who see the product frozen in its current state. Holdout group can help measure the combined effect of all product development and establish a causal relationship between the implemented changes and a company’s performance.
In doing so, one will spot weak points and highlight the strong ones more easily. The holdout group is also a powerful tool to detect issues within the A/B testing system, even after a scaled experiment.
It can be tempting to test every possible new feature added before it is rolled out. While that makes up for a tremendous data-driven mindset, the first question one must ask is: “How can this feature help grow the product/business?” The most prominent companies in the world only scale positive and statistically significant experiments: Slack (30 per cent), Microsoft (33 per cent), Bing-Google (10-20 per cent), and Netflix (10 per cent).
By fixating on a selectivity rate, one can ensure that the product does not become unnecessarily complex over time, only scaling features that bring value to its end users. The best approach is to ensure that the scale ratio is higher than the usual set p-value. This helps prevent a development team from developing dozens of unnecessary features.
Just like people can be biased, sometimes in A/B testing, if a developer sees negative results from a test, he might consider tricking the system. This can come from changing the minimum detectable effect (MDE), extending the running time, or changing the p-value, all to sway the results into the insignificant zone. Because people are subjective, good data-driven organisations must provide frameworks that remove the possibility of bias.
Another concern while performing A/B testing is how to split sample size into the correct groups for successful A/B testing results. While most sample splits are done randomly, they correlate with factors such as age, gender, or location.
For successful A/B testing, both groups must be created as homogeneous groups. The use of data science can prevent the creation of testing groups based on certain factors. Among the popular methods, the intraclass correlation coefficient (or ICC) with a value close to 0 is a good and proven strategy for effective splitting.
Besides these factors, the split ratio is also essential for determining your testing group, where a 50:50 split is the most popular and practical choice for A/B testing.
A/B Testing is a performance testing method that should be part of every data-driven organisation’s design and optimisation process. Even a slightly wrong decision can foul what would otherwise be a winning design, losing majorly on performance issues alone.
If you liked reading this, you might like our other stories
Reducing Carbon Footprint In Last-Mile Delivery
Can Data Analytics Give Your Business A Competitive Edge?