Large language models have become a dominant trend in AI, because of the massive neural network possessing infinite potential. Since OpenAI released its language model GPT-3 in 2020, the big research labs of Google, Meta, Microsoft, as well as a handful of other promising firms around the globe, have all built AIs that can generate convincing real-time text, interact with humans and answer questions, and do much more.
Recently, Alphabet’s Deepmind presented three new studies on large language models, all on the same day. The research resulted in an AI-enhanced external memory, with a vast database containing numerous passages of text, which it makes use of to generate human-like new sentences.
The Current Scenario
Language models usually can generate new text by predicting what words might come next in a sentence or a conversation. The larger a language model, the more information it can learn and process during training, which makes predictions better than traditional transformer models. GPT-3 currently possesses 175 billion parameters, values in a neural network that store data and get adjusted accordingly as the model learns from the data. Microsoft’s Megatron, the Turing language model has 530 billion parameters. But these large models also come with a huge drawback, these models take vast amounts of computing power to train, putting them out of reach in research for all but the rich organisations.
Deepmind’s NLP Revolution
Named as Retrieval-Enhanced Transforme” or RETRO, the newly developed AI-enhanced auto regressive language model comes par to the performance of neural networks that are 25 times greater in size and can help reduce the time and cost required to train very large models. The research team claims that the new database makes it easier to analyse and generate insights on what the present AI has learned, which in turn could aid in detection and filtering out bias and toxic language.
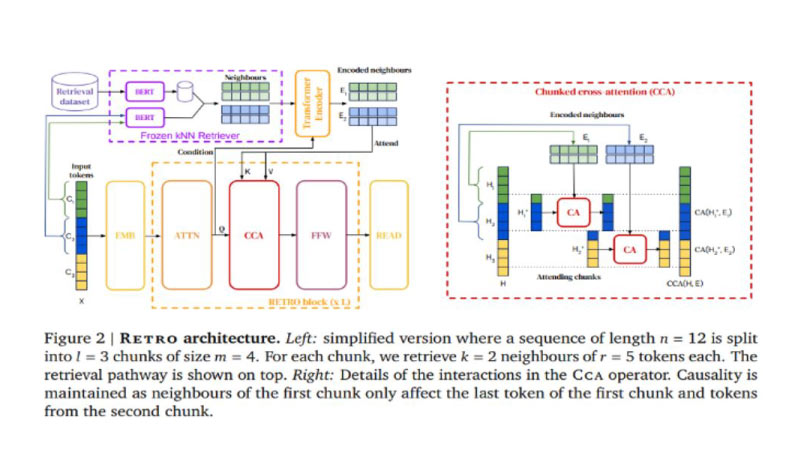
Deepmind claims that the RETRO is capable of providing a constant gain for models ranging between 150M to 7B parameters, and can be further improved at evaluation time by increasing its database size and the number of retrieved neighbours within the data.
The model has shown state-of-the-art results, on a range of evaluation datasets including 2017’s Wikitext103 and 2020’s infamous Pile, where it outperformed previous models trained on large-scale datasets and multiple baseline models at all leakage levels during the test.
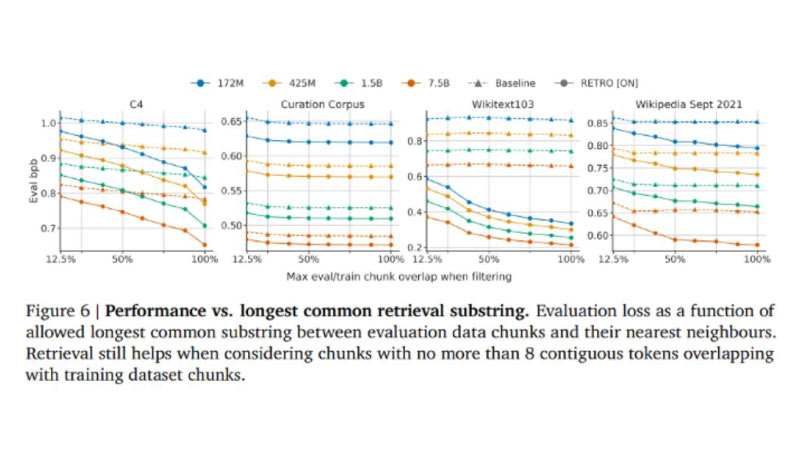
With RETRO, DeepMind has tried to also minimise the costs of training without cutting on how much the AI learns. The company says that it trained RETRO on a vast set of news articles, endless Wikipedia pages, books, and text from the online code repository GitHub. The dataset contains text in ten different languages, including English, Spanish, German, French, Russian, Chinese, Swahili, and Urdu. RETRO can be fine-tuned to achieve competitive performance on several downstream tasks such as question answering in real time, enabling the creation of futuristic chatbots.
What’s Different?
RETRO’s revolutionary new neural network has only 7 billion parameters. But the system itself contains a database consisting of nearly 2 trillion passages of text. The neural network and database are trained at the same time in RETRO, which makes it much faster than the other language model we currently have. It uses its own database during text generation to look up and compare passages similar to the one it is writing, which makes its predictions highly accurate. Outsourcing the neural network’s memory to the database during processing helps deliver more with less effort.
Is Bigger Always Better?
Deepmind’s ethics study, where a comprehensive survey of well-known problems that are inherent in large language models was carried out, showed that such transformer models pick up biases, misinformation, and toxic language such as hate speech from the articles and books they are trained on. As a result, models at times spit out harmful statements, mindlessly replicating what they encountered during the training without knowing what exactly the generated text means. Although DeepMind claims that RETRO could help address this issue, as it becomes easier to analyse what the AI has learned by examining the database than by studying the neural network, the company has not yet tested this claim.
Laura Weidinger, a research scientist at DeepMind, said: “It’s not a fully resolved problem, and work is ongoing to address these challenges.” Gen-next transformer systems like RETRO are more transparent than the usual black-box models like GPT-3, but other researchers have commented that no model can guarantee that it will prevent toxicity and bias. But still, systems like RETRO might just help raise the bar for transformer models, as it is easier to adopt language guidelines when a model makes use of external data for its predictions.
DeepMind might just be late to the ongoing debate, but it is coping up with the market requirements presenting an alternative model approach. With RETRO’s prowess, businesses can develop brand awareness through market research to generate actionable insights, implementing technologies such as sentiment analysis, real-time chatbots, and auto report generators. Such semi-parametric approaches in the near future definitely seem to provide for a more efficient method than the current raw parameter scaling for enhancing language models.
If you liked reading this, you might like our other stories