The modern data stack is immensely capable but comes with a price — complexity. Today, the stack consists of layers of abstractions comprising best of breed technologies and approaches. This layered approach provides an essential decoupling allowing architects to switch to newer and more advanced options.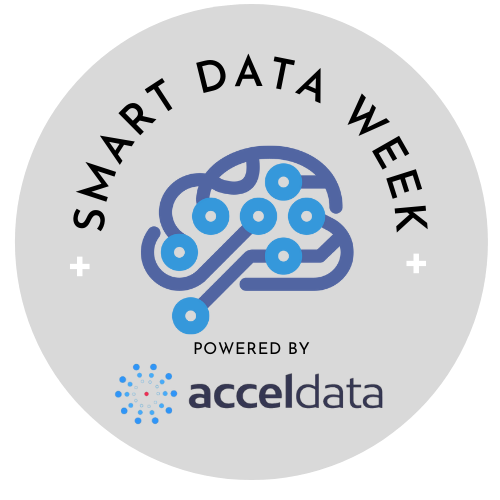
Figure 1 shows various layers of the modern data management and operations stack. What used to be a monolithic stack, now consists of multiple layers of abstraction. The new decoupled paradigm with best of breed products is leading to unprecedented innovation but, at the same time, an unintended consequence is that it is easy to lose sight of the data’s lineage and transparency.
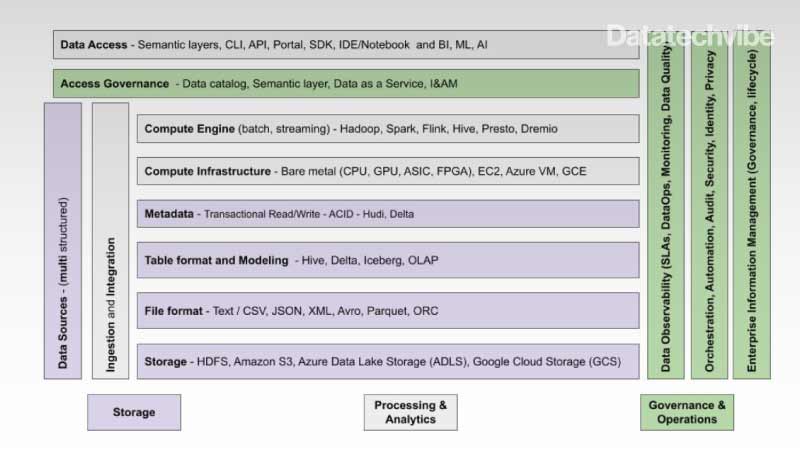
This stack is a huge improvement over the traditional approach, where every time new users or use cases were introduced, the easiest thing to do was to replicate the data required by the users. The new approach prefers to use ‘data pipelines’ to move data from the point of origin to the point of consumption, using underlying compute frameworks, which need to be monitored for performance, scale, and efficiency. The data that flows through these pipelines needs to be monitored for loss of quality. The pipelines represent the business processes that need to be monitored for SLAs.
With the traditional approach, data teams lose track of the plot after a while, and the source of truth ceases to be clear. So, we are left with an ecosystem littered with duplicate and inconsistent data. It is no surprise that data teams spend 80% of their time discovering, wrangling and munging the data, rather than analyzing the data to meet strategic goals. Data engineers, the company’s most precious resources, are working on operational issues rather than building revenue generating business use-cases.
Data observability has risen to be the all-important glue in this complex, interconnected, multi-technology world.
Also Read: 5 Challenges That Data Pipelines Must Solve
Why Data Observability?
Complexity of modern data pipelines, compute frameworks, and expanding data needs are putting unprecedented pressures on the data producers and consumers. Competitive differentiation mandates that enterprises provide their customers with excellent user experiences such as personalisation and real-time recommendations. Data therefore has become the raw material on which the business is getting built.
Data observability provides a common framework to connect disparate enterprise teams ranging from business and operations , to engineering. It allows them to create the holistic view that is mandatory to move fast and respond to business challenges in real-time.
IT teams today are stitching together silos of information across complicated and fragile data pipelines. The chasm between the business and IT leads to a lack of understanding about the data context and dependencies. A change in a source database can disrupt operations in downstream applications without any advance warning. The root cause analysis is so cumbersome that it costs not just time and money but can damage reputation and reduce trust in a brand.
On the other hand, the business teams, over the years, created their own silos and data processing environments independently. Imagine a situation where a healthcare provider’s claims team has no idea what the clinical operations or pharmacy teams are doing. If the data privacy regulations require them to forget customer information, they have to embark on an expensive and time consuming effort to resolve customer identities. This redundancy of data depletes data consistency, hurts data quality and leads to poor outcomes. Instead of concentrating resources on adding new functionality, businesses get bogged down trying to triage data availability and quality issues.
The modular stack shown in figure 1 is state of the art but it requires specialized technical skills with acute knowledge of the business processes to successfully administer the solution. IT should reduce the time spent firefighting daily operational issues and instead work on building and scaling pipelines to meet new fast-growing business requirements.
Traditionally, application monitoring tools have been deployed but these fall short as they are designed to help visualize the state of single-threaded monolithic or microservices based applications. What is needed is not more data, but precise insights. Insights should help predict and eventually prevent the operational and data availability issues by continuously monitoring and correlating the signals generated from the infrastructure, data, and application layers at scale algorithmically.
Monitoring tools build in alerts and notifications, but this is done after the fact.
- Preventing showstopper incidents through self-healing and self-tuning capabilities
- Reducing mean time to resolution for severe outage causing issues
- Increasing productivity of the operational team by removing repetitive, administrative tasks through automation
- Increasing productivity of business teams through faster development and deployment cycles
- Reducing infrastructure costs while increasing utilization of resources. This reduces overprovisioned software licenses and high costs due to misconfiguration
- Improving business ROI by eliminating unplanned outages
This is an impressive list of benefits, which are possible due to advancements in data observability platforms. However, this is only possible when the platform has a comprehensive scope.
Also Read: Why Does Data Observability Matter?
Multi-Dimensional Data Observability Scope
The scope of data observability has been interpreted differently by the vendors who are operating in this space due the focus they put on their strengths and ignore the areas where they have a weakness. For example, many data observability vendors offer a subset of functionality pertaining to simply monitoring for data quality.
A multi-dimensional data observability platform should look at a wide range of characteristics pertaining to data, data pipelines, and the infrastructure they run on. It is only through this interplay that a true picture of how the data is behaving end-to-end emerges.
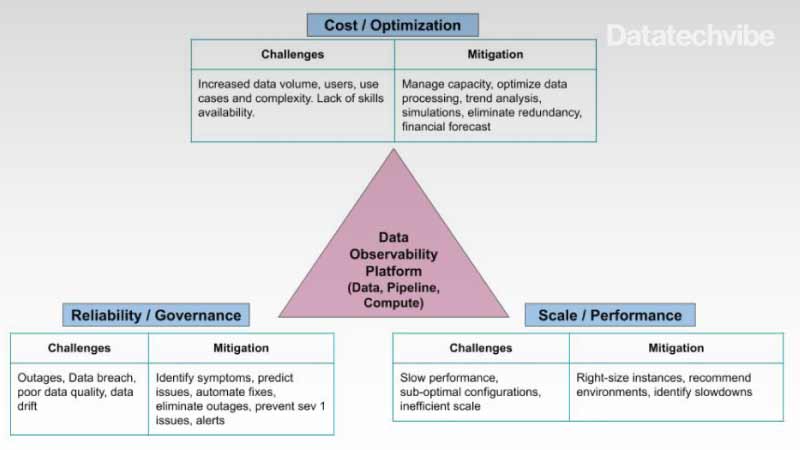
Figure 2 shows how a data observability platform should alleviate challenges related to three biggest pain points of modern data stacks which include:
- Optimising cost
- Ensuring reliability and governance
- Delivering performance and scale
A modern data observability platform takes advantage of machine learning algorithms to perform its tasks. These platforms also need connectors to the most common products that comprise the modern data stack so that they can collect and analyze the metadata and make recommendations.
Conclusion
Data is exploding in the enterprise. Businesses have an opportunity to derive more intelligence, provide better customer experience and monetize the data. This requires the modern data and analytics architecture to effectively manage all its assets, understand the flows and behaviours and proactively mitigate risks.
Just like a good mapping software is needed when planning a trip to a new destination, a data observability platform is needed to navigate the complexities of modern data architectures. The reason is that data is always changing and hence its impacts on the behavior of the pipeline. This necessitates a thorough evaluation of data observability products to ensure they meet the current and future needs of your data-driven transformation.
Multi-dimensional data observability is a requirement for enterprises to achieve significant ROI on their technology investments.
*Sanjeev Mohan, a thought leader in the areas of cloud, big data, and analytics, started his data and analytics journey at Oracle, and until recently was a research vice president at Gartner.